A mediating variable explains the process or mechanism through which one variable influences another variable. A moderating variable impacts the strength or direction of the relationship between two variables.
What is the difference between moderating and mediating variables?
The difference between mediating and moderating variables lies in their respective functions and how they influence the relationship between variables.
Attribute | Mediator Variable | Moderator Variable |
Function | Explains the process/mechanism through which the independent variable influences the dependent variable. | Affects the strength or direction of the relationship between the independent and dependent variables. |
Role | Accounts for the relationship between the independent and dependent variables, providing insight into the underlying process. | Modifies or alters the relationship between the independent and dependent variables based on different levels or conditions. |
Representation | Lies on the causal pathway between the independent and dependent variables. | Interacts with the independent variable to influence the dependent variable. |
Interpretation | Explains how or why two variables are related. | Specifies when or for whom the relationship between two variables holds or changes. |
Statistical Analysis | Mediation analysis is used to test the mediating effect. | Moderation analysis is used to test the moderating effect, often involving interaction terms. |
Mediating variables
What is a mediator variable? A mediator is how an independent variable affects a dependent variable. It is part of the causal chain leading to an effect and demonstrates how or why the effect occurs.
For a variable to be considered a mediator:
- It is caused by the independent variable.
- It causes an influence on the dependent variable.
- When accounted for, it increases the statistical correlation between the independent and dependent variables compared to when it is not included.
Mediation analysis statistically tests if a variable mediates using linear regression or ANOVA methods. Full mediation means the mediator completely explains the relationship between the independent and dependent variables; without the mediator, no relationship exists.
Partial mediation indicates a statistical relationship remains between the independent and dependent variables after removing the mediator from the model; the mediator only partially explains their relationship.
Example OF Mediator variables
Let’s consider a study investigating the relationship between job stress (independent variable) and employee burnout (dependent variable) in an organization. Researchers might hypothesize that job stress influences employee burnout through the mediating variable of sleep quality.
In this scenario, job stress affects sleep quality, which in turn affects employee burnout. Employees who experience high levels of job stress are more likely to have poor sleep quality, and those with poor sleep quality are more susceptible to burnout.
The mediating effect can be represented as follows:
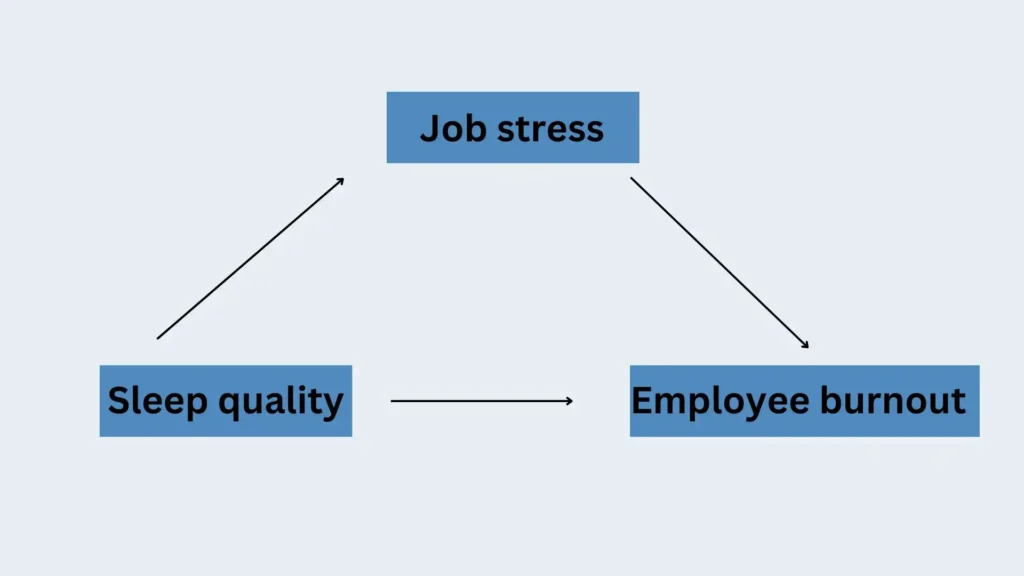
Job Stress (Independent Variable) -> Sleep Quality (Mediator Variable) -> Employee Burnout
Based on the job stress, sleep quality, and employee burnout example, if you used a descriptive research design and collected data on these variables, you would perform statistical analysis to check whether:
- Job stress predicts or is related to poor sleep quality (establishing that the independent variable influences the proposed mediator).
- Poor sleep quality predicts or is related to higher employee burnout (establishing that the proposed mediator influences the dependent variable).
- The relationship between job stress and employee burnout is stronger/larger when sleep quality is not included in the statistical model, compared to when it is included (testing for mediation).
Moderating variables
A moderator variable affects the strength or direction of the relationship between an independent variable and a dependent variable. It indicates for whom, when, or under what conditions a particular relationship exists.
Moderators help evaluate the external validity and generalizability of study findings by delineating the boundaries around when a relationship between variables holds true. For example, social media use may predict loneliness, but this relationship could be stronger for adolescents compared to older adults. In this case, age acts as a moderator.
Moderator variables can take the form of:
- Categorical variables like ethnicity, race, religion, favorite color, health status, or stimulus type.
- Quantitative variables such as age, weight, height, income, or size of a visual stimulus.
Including moderators in analyses allows researchers to specify the particular circumstances that influence whether and how strongly an effect occurs across different values or levels of the moderator variable. This enhances understanding of the limitations and scope of application for the findings.
what is the relationship between moderators and external validity?
Moderators influence the relationship between independent and dependent variables, while external validity concerns the generalizability of research findings to other contexts. By identifying moderators, researchers can better understand the conditions under which their results hold, thereby enhancing external validity. Moderators help delineate the boundaries of a study’s applicability, revealing how context-specific the findings are and improving the ability to generalize results to different populations or settings.
Example: Moderator variables
Consider a study examining the relationship between hours spent exercising per week (independent variable) and weight loss (dependent variable) among adults trying to lose weight. The researchers hypothesize that the type of diet (moderator variable) moderates the relationship between exercise and weight loss.
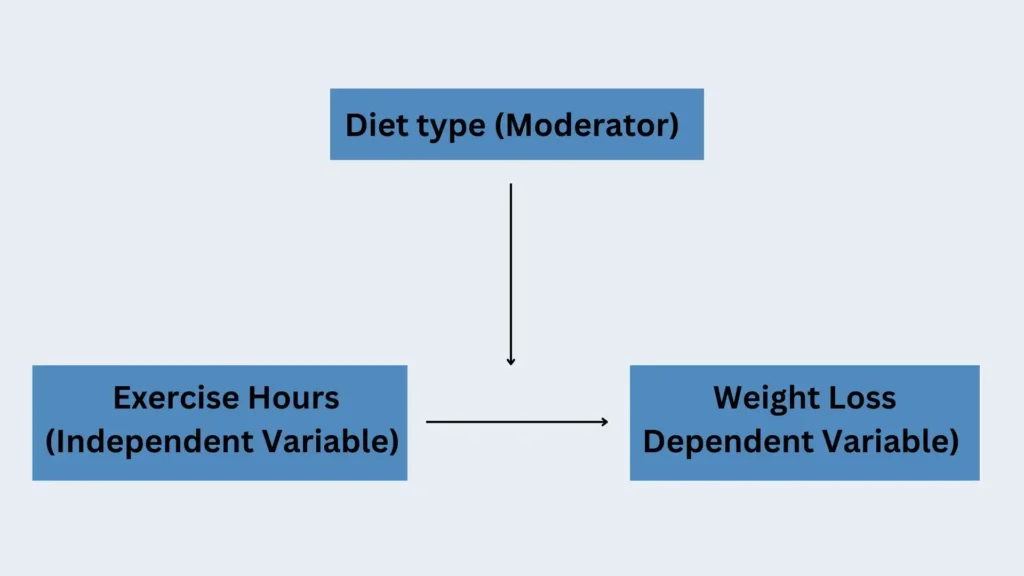
Specifically, they propose that for individuals following a low-carb diet, the relationship between exercise and weight loss will be stronger compared to those on a low-fat diet or no specific diet at all.
The prediction is that individuals spending more hours exercising will experience greater weight loss, but this effect will be amplified for people following a low-carb diet regimen.
To test this statistically, the researchers would likely employ moderation analysis using multiple regression techniques:
- Code the diet type into two dummy variables (e.g., low-carb = 1, else = 0; low-fat = 1, else = 0).
- Enter exercise hours, the two diet dummy variables, and the interaction terms (exercise x low-carb and exercise x low-fat) into a regression model predicting weight loss.
- A significant interaction between exercise hours and the low-carb diet dummy variable would indicate a moderating effect of diet type on the exercise-weight loss relationship.
This analysis would shed light on whether and how the type of diet plan someone follows impacts the degree to which exercise predicts weight loss success. A significant moderating effect suggests the exercise-weight loss connection varies in strength across diet types.
what is the difference between moderating and intervening variables?
Moderating and intervening variables are important concepts in research, but they play different roles in explaining the relationship between variables.
Aspect | Moderating Variables | Intervening Variables |
Function | Explain the process of relationship | Explain the process of the relationship |
Role | Change how a relationship works | Explain why a relationship exists |
Relationship to IV/DV | Interact with IV to influence DV | Caused by IV and cause DV |
Explanation | Don’t explain why/how IV affects DV | Mediate relationship between IV and DV |
Effect | When or for whom an effect occurs | How or why an effect occurs |
Nature | Can be qualitative or quantitative | Typically part of a causal chain |
Statistical Analysis | Often analyzed as interaction effects | Typically tested through mediation analysis |
Example | Gender moderating effect of stress on performance | Motivation intervening between goal-setting and performance |
IV = Independent Variable, DV = Dependent Variable
The mediator vs moderator debate highlights their different roles in causal pathways. While mediating vs moderating variable concepts may seem similar, they serve distinct purposes in statistical models. The moderator vs mediator comparison is often a source of confusion for new researchers. Consider mediator vs moderator examples: stress might moderate the relationship between workload and performance, while job satisfaction might mediate it.
Understanding the moderating variable vs mediating variable distinction is essential for proper research design. Researchers should be familiar with examples of mediating variables, such as attitudes mediating the effect of persuasion on behavior. In statistical analysis, the mediator vs moderator statistics approaches differ significantly.
Mediation vs moderation statistics require different analytical techniques, with mediation vs moderation analysis often employing distinct regression models. Recognizing mediating and moderating variable effects can provide a more comprehensive understanding of complex relationships. The choice between a moderator variable vs mediator variable approach depends on the research question and theoretical framework.
What are moderator variables?
Moderator variables are factors that influence the strength or direction of the relationship between an independent variable and a dependent variable. They affect when or for whom a particular relationship holds. For example, gender might moderate the relationship between stress and job performance, where the effect of stress on performance differs for men and women.
What is a mediating variable?
A mediating variable, or intervening variable, explains how an independent variable influences a dependent variable. It acts as a link in the causal chain between the two. For instance, in the relationship between exercise and mental health, stress reduction could be a mediating variable – exercise leads to stress reduction, improving mental health.