Cluster sampling is a powerful sampling method that can be particularly useful when the population you want to study is spread over a large geographical area.
Unlike stratified sampling or systematic sampling, where individual participants are selected, the cluster sampling method involves selecting entire groups or “clusters” of the population. From these groups, researchers can then select a random sample for data collection.
This probability sampling method can be more efficient and cost-effective, especially in large-scale studies or market research, as data is only collected from a subset of the clusters rather than the entire population.
For added precision, researchers sometimes use two-stage cluster sampling, where they first select the entire cluster and then randomly sample individuals within those clusters. However, while cluster sampling can save time and resources, it does come with trade-offs in terms of precision compared to other methods.
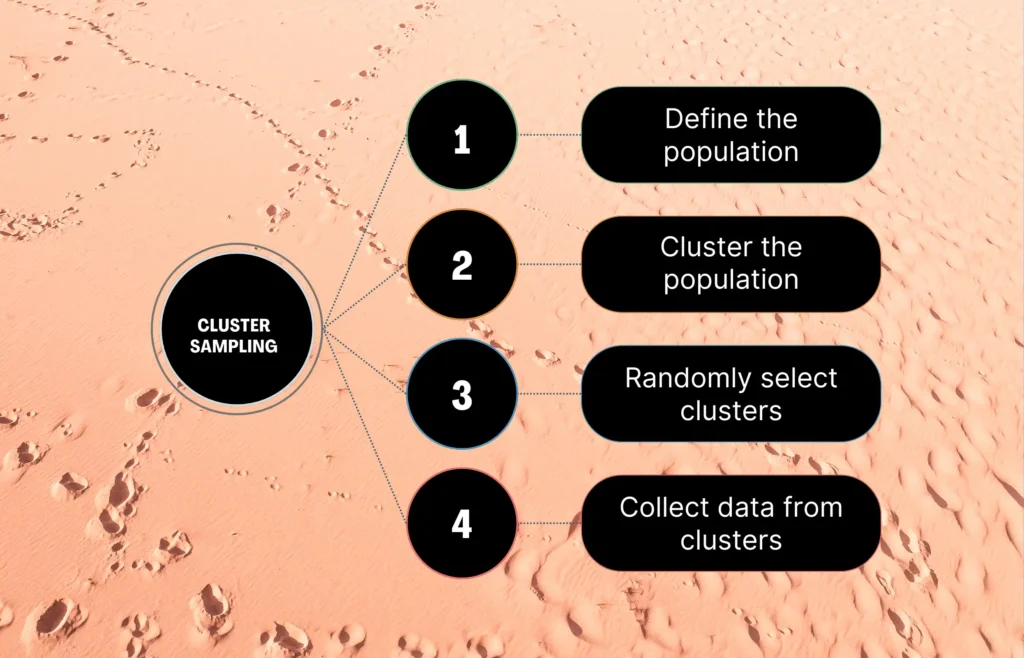
Types of Cluster Sampling
Cluster sampling is a sampling method where the researcher divides the population into groups or clusters and then selects entire clusters for the study. This type of sampling is commonly used when the population is large and spread over a wide area. The cluster sampling method offers several variations, each with its own approach to sampling. Here are the different types of cluster sampling:
Single-Stage Cluster Sampling
In single-stage cluster sampling, the researcher randomly selects entire clusters and includes all subjects within a cluster for the study. This method is simple because once the clusters are selected, no further sampling is needed within them.
An example of cluster sampling could involve selecting schools as clusters and including all students in those schools in the study. This sampling is a method that is less time-consuming, but it may introduce sampling bias due to the lack of diversity within the clusters.
Two-Stage Cluster Sampling
Two-stage sampling adds an extra step to the process. After selecting the clusters, the researcher then uses simple random sampling or systematic sampling to choose participants from within the selected clusters. This two-stage cluster sampling approach helps reduce bias in cluster sampling by increasing the diversity of participants.
For example, after selecting a random sample of schools, the researcher would then randomly select students from each school rather than including all students.
Multiple-Stage Cluster Sampling
Multiple-stage cluster sampling (or multi-stage sampling) involves more than two levels of sampling. This method uses a combination of random sampling techniques to select clusters and then sub-sample within those clusters multiple times.
For example, you could first select a geographical cluster, such as cities, then sample neighborhoods within those cities, and finally sample households within the neighborhoods. This approach can reduce cost and effort, but it also risks increasing sampling bias due to multiple stages of selection. Multi-stage sampling is a flexible approach, commonly used in large-scale surveys.
Each of these cluster sampling techniques has its advantages and trade-offs in terms of time, cost, and precision. Cluster sampling is generally considered a probability sampling method and can be useful in a variety of research contexts, but understanding the difference between cluster sampling and stratified sampling or systematic sampling vs cluster sampling is key to choosing the best approach for your study.
How to Cluster Sample
Single-stage cluster sampling, the most basic type of cluster sampling, consists of four main steps:
- Define your population
- Divide your sample into clusters
- Randomly select clusters to use as your sample
- Collect data from the sample
Research example
Suppose you want to study the reading habits of students in a large school district. Instead of randomly sampling individual students from all schools, you employ single-stage cluster sampling. You first divide the population into clusters, which are the individual schools within the district. Then, you randomly select a sample of schools (clusters) from the population. All students within the selected schools are included in your final sample, and you collect data on their reading habits from each student in these schools.
Step 1: Define your population
Before beginning the sampling process, it is essential to clearly define the population you want to study. The population consists of all the elements or individuals that share a common characteristic or set of characteristics relevant to your research question.
This could include people, objects, events, or any other entity you wish to investigate. Ensuring that your population is well-defined helps to establish the scope of your study and makes it easier to identify appropriate clusters in the next step.
Step 2: Divide your sample into clusters
Once you have defined your population, the next step is to divide it into distinct, non-overlapping subgroups called clusters. Clusters should be heterogeneous within themselves, meaning that the elements within each cluster should be diverse and representative of the entire population.
However, when compared, the clusters should be homogeneous, ensuring that each cluster is relatively similar regarding the studied characteristics. Examples of clusters include schools within a district, city households, or company departments.
Step 3: Randomly select clusters to use as your sample
After dividing the population into clusters, you will randomly select a sample of clusters to include in your study. This selection process is typically done using a probability sampling technique, such as simple random sampling, where each cluster has an equal chance of being chosen.
The number of clusters selected depends on various factors, including the desired sample size, the variability within and between clusters, and the resources available for the study. It is important to select an adequate number of clusters to ensure that the sample is representative of the population.
Step 4: Collect data from the sample
In the final step of single-stage cluster sampling, you will collect data from all the elements within each selected cluster. This means that every individual or element in the chosen clusters will be included in your final sample, and you will gather data from each.
The data collected from the elements in the sampled clusters will be used to make inferences and draw conclusions about the entire population. Ensuring that the data collection process is standardized and consistent across all selected clusters is crucial to minimize bias and errors in the results.
Multistage Cluster Sampling
In some cases, you may use a multistage clustering approach. This involves dividing the population into larger primary clusters, then randomly selecting a subset of those primary clusters and dividing them further into smaller secondary clusters from which you’ll collect data.
For example, say you’re studying a national student population. You could randomly select a few states as primary clusters, then randomly choose school districts within those states as secondary clusters, and finally randomly sample students from within the selected school districts.
Multistage cluster sampling can be beneficial when the primary clusters are large or spread out geographically. It allows you to narrow your focus in a structured way, making data collection more efficient.
Example: Multistage Sampling
Suppose you want to study job satisfaction among healthcare employees across a large country. You use multistage sampling to efficiently collect data:
- Divide the country into regions and randomly select a sample of cities within each region.
- Randomly select a sample of healthcare facilities within the selected cities using cluster sampling.
- From the chosen healthcare facilities, randomly select a sample of employees to participate in the study.
The resulting sample covers various regions, cities, and healthcare facilities while being much smaller and easier to manage than sampling every healthcare employee in the country.
Advantages and Disadvantages of Cluster Sampling
Like any sampling technique, cluster sampling has both pros and cons to consider:
Advantages | Disadvantages |
1. Cost-effective and time-efficient, especially when the population is large and geographically dispersed. | 1. Higher sampling error compared to simple random sampling, as clusters may not be fully representative of the population. |
2. There is a higher sampling error compared to simple random sampling, as clusters may not be fully representative of the population. | 2. Cluster effects can lead to higher variability within clusters, reducing the precision of estimates. |
3. Enables the study of naturally occurring groups or clusters, which can be useful for research on social or organizational dynamics. | 3. If clusters are too homogeneous, it may lead to underestimating population variability. |
4. Allows for collecting data from all elements within selected clusters, which can provide more comprehensive information. | 4. Selecting inappropriate or irrelevant clusters can lead to biased results. |
5. Facilitates the coordination and management of fieldwork, as data collection is concentrated within selected clusters. | 5. If clusters are of unequal sizes, it may require weighted analysis to account for the differences in selection probabilities. |